Delta table streaming reads and writes
Delta Lake is deeply integrated with Spark Structured Streaming through readStream
and writeStream
. Delta Lake overcomes many of the limitations typically associated with streaming systems and files, including:
Coalescing small files produced by low latency ingest.
Maintaining “exactly-once” processing with more than one stream (or concurrent batch jobs).
Efficiently discovering which files are new when using files as the source for a stream.
Note
This article describes using Delta Lake tables as streaming sources and sinks. To learn how to load data using streaming tables in Databricks SQL, see Load data using streaming tables in Databricks SQL.
For information on stream-static joins with Delta Lake, see Stream-static joins.
Delta table as a source
Structured Streaming incrementally reads Delta tables. While a streaming query is active against a Delta table, new records are processed idempotently as new table versions commit to the source table.
The follow code examples show configuring a streaming read using either the table name or file path.
spark.readStream.table("table_name")
spark.readStream.load("/path/to/table")
spark.readStream.table("table_name")
spark.readStream.load("/path/to/table")
Important
If the schema for a Delta table changes after a streaming read begins against the table, the query fails. For most schema changes, you can restart the stream to resolve schema mismatch and continue processing.
In Databricks Runtime 12.2 LTS and below, you cannot stream from a Delta table with column mapping enabled that has undergone non-additive schema evolution such as renaming or dropping columns. For details, see Streaming with column mapping and schema changes.
Limit input rate
The following options are available to control micro-batches:
maxFilesPerTrigger
: How many new files to be considered in every micro-batch. The default is 1000.maxBytesPerTrigger
: How much data gets processed in each micro-batch. This option sets a “soft max”, meaning that a batch processes approximately this amount of data and may process more than the limit in order to make the streaming query move forward in cases when the smallest input unit is larger than this limit. This is not set by default.
If you use maxBytesPerTrigger
in conjunction with maxFilesPerTrigger
, the micro-batch processes data until either the maxFilesPerTrigger
or maxBytesPerTrigger
limit is reached.
Note
In cases when the source table transactions are cleaned up due to the logRetentionDuration
configuration and the streaming query tries to process those versions, by default the query fails to avoid data loss. You can set the option failOnDataLoss
to false
to ignore lost data and continue processing.
Stream a Delta Lake change data capture (CDC) feed
Delta Lake change data feed records changes to a Delta table, including updates and deletes. When enabled, you can stream from a change data feed and write logic to process inserts, updates, and deletes into downstream tables. Although change data feed data output differs slightly from the Delta table it describes, this provides a solution for propagating incremental changes to downstream tables in a medallion architecture.
Important
In Databricks Runtime 12.2 LTS and below, you cannot stream from the change data feed for a Delta table with column mapping enabled that has undergone non-additive schema evolution such as renaming or dropping columns. See Streaming with column mapping and schema changes.
Ignore updates and deletes
Structured Streaming does not handle input that is not an append and throws an exception if any modifications occur on the table being used as a source. There are two main strategies for dealing with changes that cannot be automatically propagated downstream:
You can delete the output and checkpoint and restart the stream from the beginning.
You can set either of these two options:
ignoreDeletes
: ignore transactions that delete data at partition boundaries.skipChangeCommits
: ignore transactions that delete or modify existing records.skipChangeCommits
subsumesignoreDeletes
.
Note
In Databricks Runtime 12.2 LTS and above, skipChangeCommits
deprecates the previous setting ignoreChanges
. In Databricks Runtime 11.3 LTS and lower, ignoreChanges
is the only supported option.
The semantics for ignoreChanges
differ greatly from skipChangeCommits
. With ignoreChanges
enabled, rewritten data files in the source table are re-emitted after a data changing operation such as UPDATE
, MERGE INTO
, DELETE
(within partitions), or OVERWRITE
. Unchanged rows are often emitted alongside new rows, so downstream consumers must be able to handle duplicates. Deletes are not propagated downstream. ignoreChanges
subsumes ignoreDeletes
.
skipChangeCommits
disregards file changing operations entirely. Data files that are rewritten in the source table due to data changing operation such as UPDATE
, MERGE INTO
, DELETE
, and OVERWRITE
are ignored entirely. In order to reflect changes in upstream source tables, you must implement separate logic to propagate these changes.
Workloads configured with ignoreChanges
continue to operate using known semantics, but Databricks recommends using skipChangeCommits
for all new workloads. Migrating workloads using ignoreChanges
to skipChangeCommits
requires refactoring logic.
Example
For example, suppose you have a table user_events
with date
, user_email
, and action
columns that is partitioned by date
. You stream out of the user_events
table and you need to delete data from it due to GDPR.
When you delete at partition boundaries (that is, the WHERE
is on a partition column), the files are already segmented by value so the delete just drops those files from the metadata. When you delete an entire partition of data, you can use the following:
spark.readStream
.option("ignoreDeletes", "true")
.table("user_events")
If you delete data in multiple partitions (in this example, filtering on user_email
), use the following syntax:
spark.readStream
.option("skipChangeCommits", "true")
.table("user_events")
If you update a user_email
with the UPDATE
statement, the file containing the user_email
in question is rewritten. Use skipChangeCommits
to ignore the changed data files.
Specify initial position
You can use the following options to specify the starting point of the Delta Lake streaming source without processing the entire table.
startingVersion
: The Delta Lake version to start from. Databricks recommends omitting this option for most workloads. When not set, the stream starts from the latest available version including a complete snapshot of the table at that moment.If specified, the stream reads all changes to the Delta table starting with the specified version (inclusive). If the specified version is no longer available, the stream fails to start. You can obtain the commit versions from the
version
column of the DESCRIBE HISTORY command output.To return only the latest changes, specify
latest
.startingTimestamp
: The timestamp to start from. All table changes committed at or after the timestamp (inclusive) are read by the streaming reader. If the provided timestamp precedes all table commits, the streaming read begins with the earliest available timestamp. One of:A timestamp string. For example,
"2019-01-01T00:00:00.000Z"
.A date string. For example,
"2019-01-01"
.
You cannot set both options at the same time. They take effect only when starting a new streaming query. If a streaming query has started and the progress has been recorded in its checkpoint, these options are ignored.
Important
Although you can start the streaming source from a specified version or timestamp, the schema of the streaming source is always the latest schema of the Delta table. You must ensure there is no incompatible schema change to the Delta table after the specified version or timestamp. Otherwise, the streaming source may return incorrect results when reading the data with an incorrect schema.
Example
For example, suppose you have a table user_events
. If you want to read changes since version 5, use:
spark.readStream
.option("startingVersion", "5")
.table("user_events")
If you want to read changes since 2018-10-18, use:
spark.readStream
.option("startingTimestamp", "2018-10-18")
.table("user_events")
Process initial snapshot without data being dropped
Note
This feature is available on Databricks Runtime 11.3 LTS and above. This feature is in Public Preview.
When using a Delta table as a stream source, the query first processes all of the data present in the table. The Delta table at this version is called the initial snapshot. By default, the Delta table’s data files are processed based on which file was last modified. However, the last modification time does not necessarily represent the record event time order.
In a stateful streaming query with a defined watermark, processing files by modification time can result in records being processed in the wrong order. This could lead to records dropping as late events by the watermark.
You can avoid the data drop issue by enabling the following option:
withEventTimeOrder: Whether the initial snapshot should be processed with event time order.
With event time order enabled, the event time range of initial snapshot data is divided into time buckets. Each micro batch processes a bucket by filtering data within the time range. The maxFilesPerTrigger and maxBytesPerTrigger configuration options are still applicable to control the microbatch size but only in an approximate way due to the nature of the processing.
The graphic below shows this process:
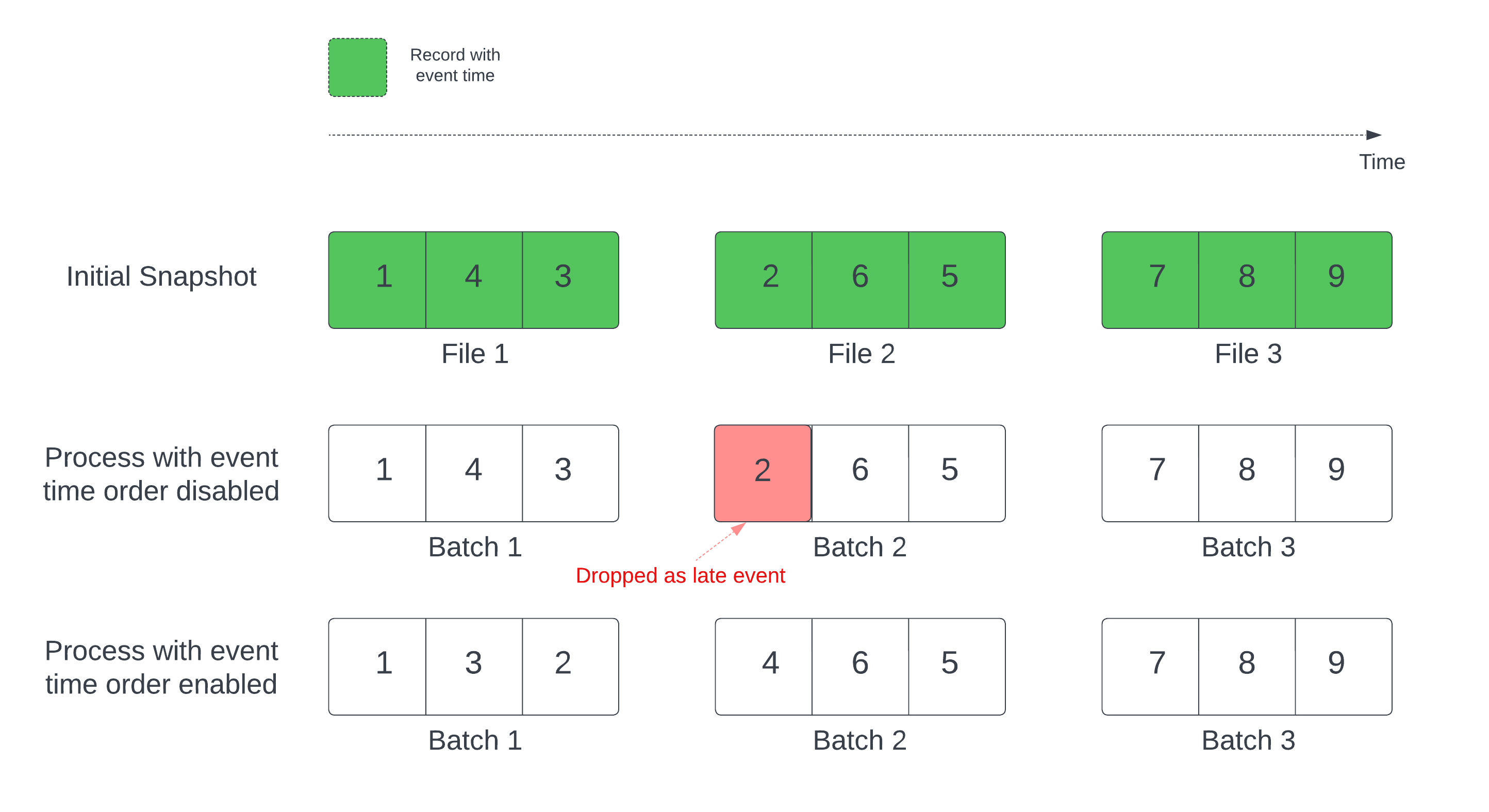
Notable information about this feature:
The data drop issue only happens when the initial Delta snapshot of a stateful streaming query is processed in the default order.
You cannot change
withEventTimeOrder
once the stream query is started while the initial snapshot is still being processed. To restart withwithEventTimeOrder
changed, you need to delete the checkpoint.If you are running a stream query with withEventTimeOrder enabled, you cannot downgrade it to a DBR version which doesn’t support this feature until the initial snapshot processing is completed. If you need to downgrade, you can wait for the initial snapshot to finish, or delete the checkpoint and restart the query.
This feature is not supported in the following uncommon scenarios:
The event time column is a generated column and there are non-projection transformations between the Delta source and watermark.
There is a watermark that has more than one Delta source in the stream query.
With event time order enabled, the performance of the Delta initial snapshot processing might be slower.
Each micro batch scans the initial snapshot to filter data within the corresponding event time range. For faster filter action, it is advised to use a Delta source column as the event time so that data skipping can be applied (check Data skipping for Delta Lake for when it’s applicable). Additionally, table partitioning along the event time column can further speed the processing. You can check Spark UI to see how many delta files are scanned for a specific micro batch.
Example
Suppose you have a table user_events
with an event_time
column. Your streaming query is an aggregation query. If you want to ensure no data drop during the initial snapshot processing, you can use:
spark.readStream
.option("withEventTimeOrder", "true")
.table("user_events")
.withWatermark("event_time", "10 seconds")
Note
You can also enable this with Spark config on the cluster which will apply to all streaming queries: spark.databricks.delta.withEventTimeOrder.enabled true
Delta table as a sink
You can also write data into a Delta table using Structured Streaming. The transaction log enables Delta Lake to guarantee exactly-once processing, even when there are other streams or batch queries running concurrently against the table.
Note
The Delta Lake VACUUM
function removes all files not managed by Delta Lake but skips any directories that begin with _
. You can safely store checkpoints alongside other data and metadata for a Delta table using a directory structure such as <table-name>/_checkpoints
.
Metrics
You can find out the number of bytes and number of files yet to be processed in a streaming query process as the numBytesOutstanding
and numFilesOutstanding
metrics. Additional metrics include:
numNewListedFiles
: Number of Delta Lake files that were listed in order to calculate the backlog for this batch.backlogEndOffset
: The table version used to calculate the backlog.
If you are running the stream in a notebook, you can see these metrics under the Raw Data tab in the streaming query progress dashboard:
{
"sources" : [
{
"description" : "DeltaSource[file:/path/to/source]",
"metrics" : {
"numBytesOutstanding" : "3456",
"numFilesOutstanding" : "8"
},
}
]
}
Append mode
By default, streams run in append mode, which adds new records to the table.
Use the toTable
method when streaming to tables, as in the following example:
(events.writeStream
.outputMode("append")
.option("checkpointLocation", "/tmp/delta/events/_checkpoints/")
.toTable("events")
)
events.writeStream
.outputMode("append")
.option("checkpointLocation", "/tmp/delta/events/_checkpoints/")
.toTable("events")
Complete mode
You can also use Structured Streaming to replace the entire table with every batch. One example use case is to compute a summary using aggregation:
(spark.readStream
.table("events")
.groupBy("customerId")
.count()
.writeStream
.outputMode("complete")
.option("checkpointLocation", "/tmp/delta/eventsByCustomer/_checkpoints/")
.toTable("events_by_customer")
)
spark.readStream
.table("events")
.groupBy("customerId")
.count()
.writeStream
.outputMode("complete")
.option("checkpointLocation", "/tmp/delta/eventsByCustomer/_checkpoints/")
.toTable("events_by_customer")
The preceding example continuously updates a table that contains the aggregate number of events by customer.
For applications with more lenient latency requirements, you can save computing resources with one-time triggers. Use these to update summary aggregation tables on a given schedule, processing only new data that has arrived since the last update.
Upsert from streaming queries using foreachBatch
You can use a combination of merge
and foreachBatch
to write complex upserts from a streaming query into a Delta table. See Use foreachBatch to write to arbitrary data sinks.
This pattern has many applications, including the following:
Write streaming aggregates in Update Mode: This is much more efficient than Complete Mode.
Write a stream of database changes into a Delta table: The merge query for writing change data can be used in
foreachBatch
to continuously apply a stream of changes to a Delta table.Write a stream of data into Delta table with deduplication: The insert-only merge query for deduplication can be used in
foreachBatch
to continuously write data (with duplicates) to a Delta table with automatic deduplication.
Note
Make sure that your
merge
statement insideforeachBatch
is idempotent as restarts of the streaming query can apply the operation on the same batch of data multiple times.When
merge
is used inforeachBatch
, the input data rate of the streaming query (reported throughStreamingQueryProgress
and visible in the notebook rate graph) may be reported as a multiple of the actual rate at which data is generated at the source. This is becausemerge
reads the input data multiple times causing the input metrics to be multiplied. If this is a bottleneck, you can cache the batch DataFrame beforemerge
and then uncache it aftermerge
.
The following example demonstrates how you can use SQL within foreachBatch
to accomplish this task:
// Function to upsert microBatchOutputDF into Delta table using merge
def upsertToDelta(microBatchOutputDF: DataFrame, batchId: Long) {
// Set the dataframe to view name
microBatchOutputDF.createOrReplaceTempView("updates")
// Use the view name to apply MERGE
// NOTE: You have to use the SparkSession that has been used to define the `updates` dataframe
microBatchOutputDF.sparkSession.sql(s"""
MERGE INTO aggregates t
USING updates s
ON s.key = t.key
WHEN MATCHED THEN UPDATE SET *
WHEN NOT MATCHED THEN INSERT *
""")
}
// Write the output of a streaming aggregation query into Delta table
streamingAggregatesDF.writeStream
.foreachBatch(upsertToDelta _)
.outputMode("update")
.start()
# Function to upsert microBatchOutputDF into Delta table using merge
def upsertToDelta(microBatchOutputDF, batchId):
# Set the dataframe to view name
microBatchOutputDF.createOrReplaceTempView("updates")
# Use the view name to apply MERGE
# NOTE: You have to use the SparkSession that has been used to define the `updates` dataframe
# In Databricks Runtime 10.5 and below, you must use the following:
# microBatchOutputDF._jdf.sparkSession().sql("""
microBatchOutputDF.sparkSession.sql("""
MERGE INTO aggregates t
USING updates s
ON s.key = t.key
WHEN MATCHED THEN UPDATE SET *
WHEN NOT MATCHED THEN INSERT *
""")
# Write the output of a streaming aggregation query into Delta table
(streamingAggregatesDF.writeStream
.foreachBatch(upsertToDelta)
.outputMode("update")
.start()
)
You can also choose to use the Delta Lake APIs to perform streaming upserts, as in the following example:
import io.delta.tables.*
val deltaTable = DeltaTable.forName(spark, "table_name")
// Function to upsert microBatchOutputDF into Delta table using merge
def upsertToDelta(microBatchOutputDF: DataFrame, batchId: Long) {
deltaTable.as("t")
.merge(
microBatchOutputDF.as("s"),
"s.key = t.key")
.whenMatched().updateAll()
.whenNotMatched().insertAll()
.execute()
}
// Write the output of a streaming aggregation query into Delta table
streamingAggregatesDF.writeStream
.foreachBatch(upsertToDelta _)
.outputMode("update")
.start()
from delta.tables import *
deltaTable = DeltaTable.forName(spark, "table_name")
# Function to upsert microBatchOutputDF into Delta table using merge
def upsertToDelta(microBatchOutputDF, batchId):
(deltaTable.alias("t").merge(
microBatchOutputDF.alias("s"),
"s.key = t.key")
.whenMatchedUpdateAll()
.whenNotMatchedInsertAll()
.execute()
)
# Write the output of a streaming aggregation query into Delta table
(streamingAggregatesDF.writeStream
.foreachBatch(upsertToDelta)
.outputMode("update")
.start()
)
Idempotent table writes in foreachBatch
Note
Databricks recommends configuring a separate streaming write for each sink you wish to update. Using foreachBatch
to write to multiple tables serializes writes, which reduces parallelizaiton and increases overall latency.
Delta tables support the following DataFrameWriter
options to make writes to multiple tables within foreachBatch
idempotent:
txnAppId
: A unique string that you can pass on each DataFrame write. For example, you can use the StreamingQuery ID astxnAppId
.txnVersion
: A monotonically increasing number that acts as transaction version.
Delta Lake uses the combination of txnAppId
and txnVersion
to identify duplicate writes and ignore them.
If a batch write is interrupted with a failure, re-running the batch uses the same application and batch ID to help the runtime correctly identify duplicate writes and ignore them. Application ID (txnAppId
) can be any user-generated unique string and does not have to be related to the stream ID. See Use foreachBatch to write to arbitrary data sinks.
Warning
If you delete the streaming checkpoint and restart the query with a new checkpoint, you must provide a different txnAppId
. New checkpoints start with a batch ID of 0
. Delta Lake uses the batch ID and txnAppId
as a unique key, and skips batches with already seen values.
The following code example demonstrates this pattern:
app_id = ... # A unique string that is used as an application ID.
def writeToDeltaLakeTableIdempotent(batch_df, batch_id):
batch_df.write.format(...).option("txnVersion", batch_id).option("txnAppId", app_id).save(...) # location 1
batch_df.write.format(...).option("txnVersion", batch_id).option("txnAppId", app_id).save(...) # location 2
streamingDF.writeStream.foreachBatch(writeToDeltaLakeTableIdempotent).start()
val appId = ... // A unique string that is used as an application ID.
streamingDF.writeStream.foreachBatch { (batchDF: DataFrame, batchId: Long) =>
batchDF.write.format(...).option("txnVersion", batchId).option("txnAppId", appId).save(...) // location 1
batchDF.write.format(...).option("txnVersion", batchId).option("txnAppId", appId).save(...) // location 2
}