Pythonのユーザー定義関数を使用したオンデマンドの特徴量計算
この記事では、Databricks でオンデマンド機能を作成して使用する方法について説明します。
オンデマンド機能を使用するには、ワークスペースで Unity Catalog を有効にし、 Databricks Runtime 13.3 LTS 機械学習以降を使用する必要があります。
オンデマンド特徴量とは何ですか?
「オンデマンド」とは、値が事前にわかっていないが、推論時に計算される特徴量を指します。 Databricks では、 Python ユーザー定義関数 (UDF) を使用して、オンデマンド特徴量の計算方法を指定します。 これらの関数は Unity Catalog によって管理され、 カタログエクスプローラー で検出できます。
要件
ユーザー定義関数 ( UDF ) を使用してトレーニング セットを作成したり、 Feature Servingポイントを作成したりするには、 Unity Catalogの
system
カタログに対するUSE CATALOG
権限が必要です。
ワークフロー
オンデマンドで特徴量を計算するには、特徴量の値の計算方法を記述する Python ユーザー定義関数 (UDF) を指定します。
トレーニング中に、この関数とその入力バインドを
create_training_set
API のfeature_lookups
パラメーターで指定します。トレーニング済みのモデルは、Feature Store メソッド
log_model
を使用してログに記録する必要があります。 これにより、モデルが推論に使用されるときにオンデマンド機能が自動的に評価されます。バッチ スコアリングの場合、
score_batch
API は、オンデマンド機能を含むすべての機能値を自動的に計算して返します。Mosaic AI Model Servingを使用してモデルを提供すると、モデルは自動的にPython UDFを使用して、スコアリング リクエストごとにオンデマンドの特徴を計算します。
Python UDFを作成する
Python UDF は、ノートブックまたは Databricks SQL で作成できます。
たとえば、ノートブック セルで次のコードを実行すると、カタログ main
とスキーマ default
に Python UDF example_feature
が作成されます。
%sql
CREATE FUNCTION main.default.example_feature(x INT, y INT)
RETURNS INT
LANGUAGE PYTHON
COMMENT 'add two numbers'
AS $$
def add_numbers(n1: int, n2: int) -> int:
return n1 + n2
return add_numbers(x, y)
$$
コードの実行後、「 カタログエクスプローラ」(Catalog Explorer ) で 3 レベルのネームスペース内を移動して、関数定義を表示できます。
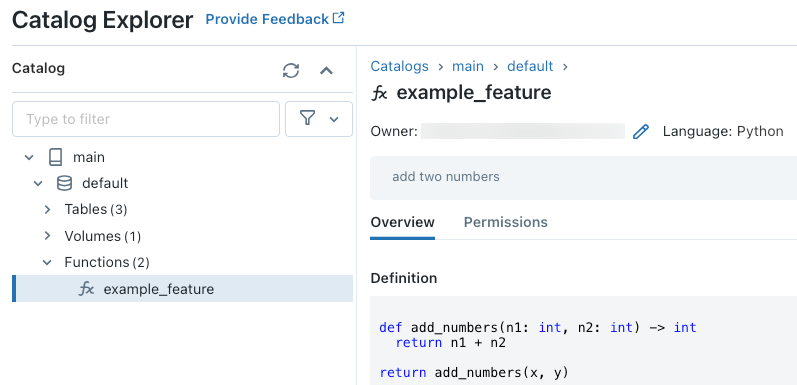
Python UDF の作成の詳細については、「 Python UDF を Unity Catalog に登録する 」と SQL 言語のマニュアルを参照してください。
欠損している特徴量の値を処理する方法
Python UDF が FeatureLookup の結果に依存する場合、要求されたルックアップ キーが見つからない場合に返される値は環境によって異なります。 score_batch
を使用する場合、返される値は None
です。オンライン配信を使用する場合、返される値は float("nan")
です。
次のコードは、両方のケースを処理する方法の例です。
%sql
CREATE OR REPLACE FUNCTION square(x INT)
RETURNS INT
LANGUAGE PYTHON AS
$$
import numpy as np
if x is None or np.isnan(x):
return 0
return x * x
$$
オンデマンド特徴量を用いてモデルをトレーニングする
モデルをトレーニングするには、 feature_lookups
パラメーターで create_training_set
API に渡される FeatureFunction
を使用します。
次のコード例では、前のセクションで定義した Python UDF main.default.example_feature
を使用しています。
# Install databricks-feature-engineering first with:
# %pip install databricks-feature-engineering
# dbutils.library.restartPython()
from databricks.feature_engineering import FeatureEngineeringClient
from databricks.feature_engineering import FeatureFunction, FeatureLookup
from sklearn import linear_model
fe = FeatureEngineeringClient()
features = [
# The feature 'on_demand_feature' is computed as the sum of the the input value 'new_source_input'
# and the pre-materialized feature 'materialized_feature_value'.
# - 'new_source_input' must be included in base_df and also provided at inference time.
# - For batch inference, it must be included in the DataFrame passed to 'FeatureEngineeringClient.score_batch'.
# - For real-time inference, it must be included in the request.
# - 'materialized_feature_value' is looked up from a feature table.
FeatureFunction(
udf_name="main.default.example_feature", # UDF must be in Unity Catalog so uses a three-level namespace
input_bindings={
"x": "new_source_input",
"y": "materialized_feature_value"
},
output_name="on_demand_feature",
),
# retrieve the prematerialized feature
FeatureLookup(
table_name = 'main.default.table',
feature_names = ['materialized_feature_value'],
lookup_key = 'id'
)
]
# base_df includes the columns 'id', 'new_source_input', and 'label'
training_set = fe.create_training_set(
df=base_df,
feature_lookups=features,
label='label',
exclude_columns=['id', 'new_source_input', 'materialized_feature_value'] # drop the columns not used for training
)
# The training set contains the columns 'on_demand_feature' and 'label'.
training_df = training_set.load_df().toPandas()
# training_df columns ['materialized_feature_value', 'label']
X_train = training_df.drop(['label'], axis=1)
y_train = training_df.label
model = linear_model.LinearRegression().fit(X_train, y_train)
モデルをログに記録し、 Unity Catalogに登録する
フィーチャ メタデータと共にパッケージ化されたモデルは 、 Unity Catalogに登録できます。モデルの作成に使用するフィーチャー テーブルは、 Unity Catalogに格納する必要があります。
モデルが推論に使用されるときにオンデマンド機能を自動的に評価するようにするには、次のようにレジストリ URI を設定し、モデルをログに記録する必要があります。
import mlflow
mlflow.set_registry_uri("databricks-uc")
fe.log_model(
model=model,
artifact_path="main.default.model",
flavor=mlflow.sklearn,
training_set=training_set,
registered_model_name="main.default.recommender_model"
)
オンデマンド機能を定義する Python UDF が Python パッケージをインポートする場合は、引数 extra_pip_requirements
を使用してこれらのパッケージを指定する必要があります。 例えば:
import mlflow
mlflow.set_registry_uri("databricks-uc")
fe.log_model(
model=model,
artifact_path="model",
flavor=mlflow.sklearn,
training_set=training_set,
registered_model_name="main.default.recommender_model",
extra_pip_requirements=["scikit-learn==1.20.3"]
)
制約
オンデマンド機能は、MapType と ArrayType を除く Feature Store でサポートされているすべてのデータ型 を出力できます。