Feature engineering and serving
This page covers feature engineering and serving capabilities for workspaces that are enabled for Unity Catalog. If your workspace is not enabled for Unity Catalog, see Workspace Feature Store (legacy).
Why use Databricks as your feature store?
With the Databricks Data Intelligence Platform, the entire model training workflow takes place on a single platform:
Data pipelines that ingest raw data, create feature tables, train models, and perform batch inference. When you train and log a model using feature engineering in Unity Catalog, the model is packaged with feature metadata. When you use the model for batch scoring or online inference, it automatically retrieves feature values. The caller does not need to know about them or include logic to look up or join features to score new data.
Model and feature serving endpoints that are available with a single click and that provide milliseconds of latency.
Data and model monitoring.
In addition, the platform provides the following:
Feature discovery. You can browse and search for features in the Databricks UI.
Governance. Feature tables, functions, and models are all governed by Unity Catalog. When you train a model, it inherits permissions from the data it was trained on.
Lineage. When you create a feature table in Databricks, the data sources used to create the feature table are saved and accessible. For each feature in a feature table, you can also access the models, notebooks, jobs, and endpoints that use the feature.
Cross-workspace access. Feature tables, functions, and models are automatically available in any workspace that has access to the catalog.
Requirements
Your workspace must be enabled for Unity Catalog.
Feature engineering in Unity Catalog requires Databricks Runtime 13.3 LTS or above.
If your workspace does not meet these requirements, see Workspace Feature Store (legacy) for how to use the legacy Workspace Feature Store.
How does feature engineering on Databricks work?
The typical machine learning workflow using feature engineering on Databricks follows this path:
Write code to convert raw data into features and create a Spark DataFrame containing the desired features.
Create a Delta table in Unity Catalog. Any Delta table with a primary key is automatically a feature table.
Train and log a model using the feature table. When you do this, the model stores the specifications of features used for training. When the model is used for inference, it automatically joins features from the appropriate feature tables.
Register model in Model Registry.
You can now use the model to make predictions on new data. For batch use cases, the model automatically retrieves the features it needs from Feature Store.
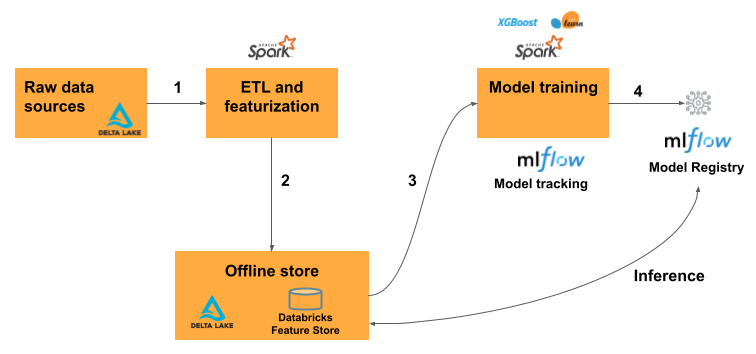
For real-time serving use cases, publish the features to an online table. Third-party online stores are also supported. See Third-party online stores.
At inference time, the model reads pre-computed features from the online store and joins them with the data provided in the client request to the model serving endpoint.
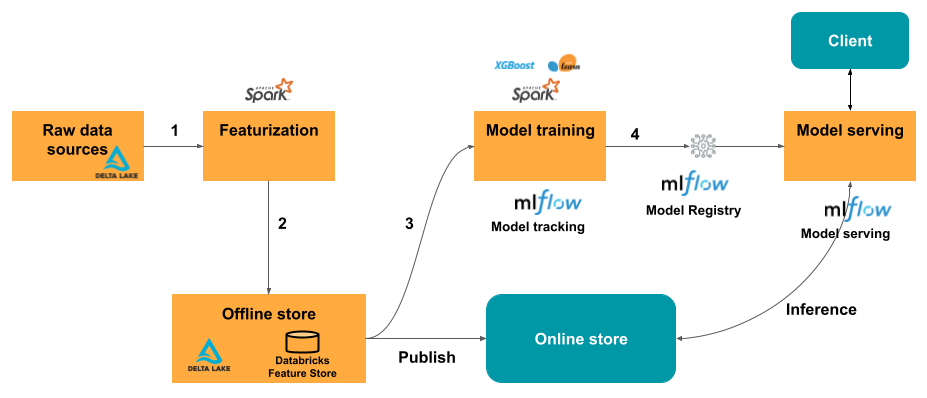
Start using feature engineering — example notebooks
To get started, try these example notebooks. The basic notebook steps you through how to create a feature table, use it to train a model, and then perform batch scoring using automatic feature lookup. It also introduces you to the Feature Engineering UI and shows how you can use it to search for features and understand how features are created and used.
The taxi example notebook illustrates the process of creating features, updating them, and using them for model training and batch inference.
Supported data types
Feature engineering in Unity Catalog and legacy Workspace Feature Store support the following PySpark data types:
IntegerType
FloatType
BooleanType
StringType
DoubleType
LongType
TimestampType
DateType
ShortType
ArrayType
BinaryType
[1]DecimalType
[1]MapType
[1]StructType
[2]
[1] BinaryType
, DecimalType
, and MapType
are supported in all versions of Feature Engineering in Unity Catalog and in Workspace Feature Store v0.3.5 or above.
[2] StructType
is supported in Feature Engineering v0.6.0 or above.
The data types listed above support feature types that are common in machine learning applications. For example:
You can store dense vectors, tensors, and embeddings as
ArrayType
.You can store sparse vectors, tensors, and embeddings as
MapType
.You can store text as
StringType
.
When published to online stores, ArrayType
and MapType
features are stored in JSON format.
The Feature Store UI displays metadata on feature data types:
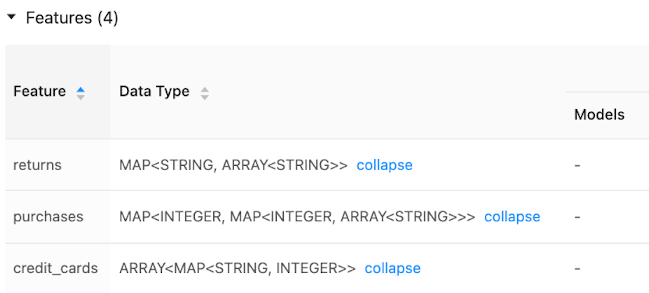
More information
For more information on best practices, download The Comprehensive Guide to Feature Stores.