AI and machine learning on Databricks
This article describes the tools that Mosaic AI (formerly Databricks Machine Learning) provides to help you build AI and ML systems. The diagram shows how various products on Databricks platform help you implement your end to end workflows to build and deploy AI and ML systems
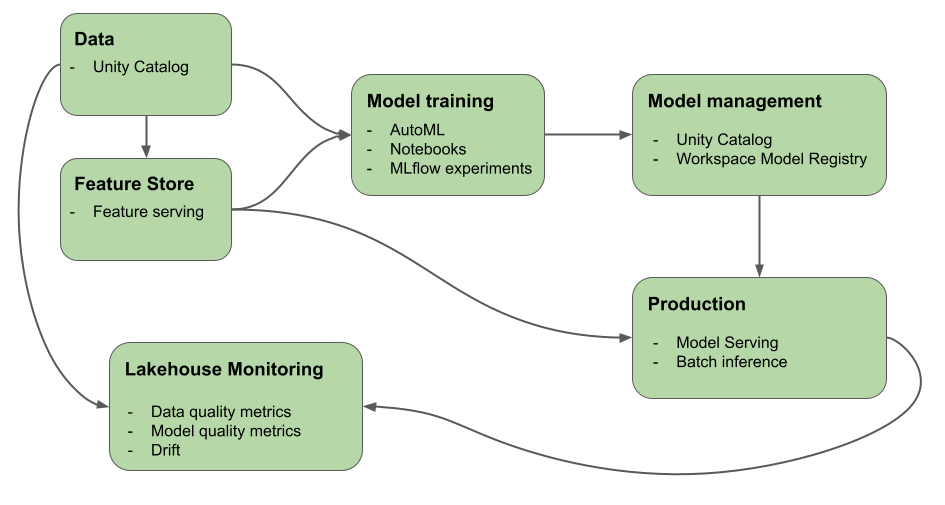
Generative AI on Databricks
Mosaic AI unifies the AI lifecycle from data collection and preparation, to model development and LLMOps, to serving and monitoring. The following features are specifically optimized to facilitate the development of generative AI applications:
Unity Catalog for governance, discovery, versioning, and access control for data, features, models, and functions.
MLflow for model development tracking.
Mosaic AI Model Serving for deploying LLMs. You can configure a model serving endpoint specifically for accessing generative AI models:
State-of-the-art open LLMs using Foundation Model APIs.
Third-party models hosted outside of Databricks. See External models in Mosaic AI Model Serving.
Mosaic AI Vector Search provides a queryable vector database that stores embedding vectors and can be configured to automatically sync to your knowledge base.
Lakehouse Monitoring for data monitoring and tracking model prediction quality and drift using automatic payload logging with inference tables.
AI Playground for testing generative AI models from your Databricks workspace. You can prompt, compare and adjust settings such as system prompt and inference parameters.
Foundation Model Fine-tuning (now part of Mosaic AI Model Training) for customizing a foundation model using your own data to optimize its performance for your specific application.
Mosaic AI Agent Framework for building and deploying production-quality agents like Retrieval Augmented Generation (RAG) applications.
Mosaic AI Agent Evaluation for evaluating the quality, cost, and latency of generative AI applications, including RAG applications and chains.
What is generative AI?
Generative AI is a type of artificial intelligence focused on the ability of computers to use models to create content like images, text, code, and synthetic data.
Generative AI applications are built on top of generative AI models: large language models (LLMs) and foundation models.
LLMs are deep learning models that consume and train on massive datasets to excel in language processing tasks. They create new combinations of text that mimic natural language based on their training data.
Generative AI models or foundation models are large ML models pre-trained with the intention that they are to be fine-tuned for more specific language understanding and generation tasks. These models are used to discern patterns within the input data.
After these models have completed their learning processes, together they generate statistically probable outputs when prompted and they can be employed to accomplish various tasks, including:
Image generation based on existing ones or utilizing the style of one image to modify or create a new one.
Speech tasks such as transcription, translation, question/answer generation, and interpretation of the intent or meaning of text.
Important
While many LLMs or other generative AI models have safeguards, they can still generate harmful or inaccurate information.
Generative AI has the following design patterns:
Prompt Engineering: Crafting specialized prompts to guide LLM behavior
Retrieval Augmented Generation (RAG): Combining an LLM with external knowledge retrieval
Fine-tuning: Adapting a pre-trained LLM to specific data sets of domains
Pre-training: Training an LLM from scratch
Machine learning on Databricks
With Mosaic AI, a single platform serves every step of ML development and deployment, from raw data to inference tables that save every request and response for a served model. Data scientists, data engineers, ML engineers and DevOps can do their jobs using the same set of tools and a single source of truth for the data.
Mosaic AI unifies the data layer and ML platform. All data assets and artifacts, such as models and functions, are discoverable and governed in a single catalog. Using a single platform for data and models makes it possible to track lineage from the raw data to the production model. Built-in data and model monitoring saves quality metrics to tables that are also stored in the platform, making it easier to identify the root cause of model performance problems. For more information about how Databricks supports the full ML lifecycle and MLOps, see MLOps workflows on Databricks and MLOps Stacks: model development process as code.
Some of the key components of the data intelligence platform are:
Tasks |
Component |
---|---|
Govern and manage data, features, models, and functions. Also discovery, versioning, and lineage. |
|
Track changes to data, data quality, and model prediction quality |
|
Feature development and management |
|
Train models |
|
Track model development |
|
Serve custom models |
|
Build automated workflows and production-ready ETL pipelines |
|
Git integration |
Deep learning on Databricks
Configuring infrastructure for deep learning applications can be difficult. Databricks Runtime for Machine Learning takes care of that for you, with clusters that have built-in compatible versions of the most common deep learning libraries like TensorFlow, PyTorch, and Keras.
Databricks Runtime ML clusters also include pre-configured GPU support with drivers and supporting libraries. It also supports libraries like Ray to parallelize compute processing for scaling ML workflows and ML applications.
Databricks Runtime ML clusters also include pre-configured GPU support with drivers and supporting libraries. Mosaic AI Model Serving enables creation of scalable GPU endpoints for deep learning models with no extra configuration.
For machine learning applications, Databricks recommends using a cluster running Databricks Runtime for Machine Learning. See Create a cluster using Databricks Runtime ML.
To get started with deep learning on Databricks, see: